Over a decade after the idea of “big data” was first born, data continues to be one of the most important and furiously growing innovation drivers across both large enterprises and new startups. From providing pulse checks that are foundational to business operations to intelligently automating daily tasks through machine learning, data has become the central nervous system for decision-making in organizations of all sizes. Moreover, the use of data now reaches well beyond data scientists, data analysts, and data engineers — everyone is a data producer and consumer.
The result of this increased focus on data: The business of managing data has already become one of the fastest growing areas of infrastructure, estimated to be worth over $70B and accounts for over one-fifth of all enterprise infrastructure spend in 2021. The beauty of this market’s formation is that it marries the field of software engineering, analytics, and artificial intelligence, while riding the tidal momentum of cloud computing. (For more on the architectural evolution and driving forces behind this massive trend, see this piece, Emerging Architectures for Modern Data Infrastructure, which was just updated for 2022.)
The growth of the data industry has also given birth to some of the most exciting and impactful enterprise software companies in the last few years. Recent public juggernauts such as Snowflake and Confluent have already changed the way thousands of businesses operate and millions of products are built. However, most people are less familiar with the movers and shakers — the next generation of category-defining companies.
To help cut through the noise after a record-breaking 2021 in which data companies received tens of billions in venture capital investment — and an already strong 2022 — we’ve compiled the inaugural class of the Data50. These are the bellwether companies across the most exciting categories in data. In aggregate, these 50 companies are valued at more than $100B and have raised approximately $14.5B in total capital, with 20 having reached unicorn status by 2021.
Without further ado, we’re excited to introduce the Data50 of 2022.
The Data50 List
Rank | Company | Category | Location | Valuation Range | Website |
---|
1 | ![]() | Query and Processing | San Francisco, CA | $5B+ | Databricks |
2 | ![]() | ELT & Orchestration | Oakland, CA | $5B+ | Fivetran |
3 | ![]() | AI/ML | Palo Alto, CA | $5B+ | Scale.ai |
4 | ![]() | Data Governance & Security | Atlanta, GA | $5B+ | OneTrust |
5 | ![]() | ELT & Orchestration | Philadelphia, PA | $1B-$5B | Dbt labs |
6 | ![]() | Query and Processing | Boston, MA | $1B-$5B | Starburst |
7 | ![]() | Data Governance & Security | Brussels, Belgium | $5B+ | Collibra |
8 | ![]() | Query and Processing | Santa Clara, CA | $1B-$5B | Dremio |
9 | ![]() | Query and Processing | New York, NY | $1B-$5B | Dataiku |
10 | ![]() | AI/ML | New York, NY | $250-999M | Hugging Face |
11 | ![]() | Query and Processing | Boston, MA | $5B+ | DataRobot |
12 | ![]() | AI/ML | San Francisco, CA | $250-999M | Primer.ai |
13 | ![]() | AI/ML | Palo Alto, CA | $1B-$5B | Snorkel |
14 | ![]() | AI/ML | San Francisco, CA | $1B-$5B | Anyscale |
15 | ![]() | Query and Processing | Tel Aviv, Israel | $1B-$5B | Firebolt |
16 | ![]() | ELT & Orchestration | Cincinnati, OH | $100-$249M | Astronomer |
17 | ![]() | Data Governance & Security | Redwood City, CA | $1B-$5B | Alation |
18 | ![]() | AI/ML | San Francisco, CA | $1B-$5B | Weights & Biases |
19 | ![]() | BI & Notebooks | San Francisco, CA | $1B-$5B | Sigma Computing |
20 | ![]() | Data Observability | San Francisco, CA | $250-999M | Monte Carlo |
21 | ![]() | AI/ML | Seattle, WA | $250-999M | OctoML |
22 | ![]() | Customer Data Analytics | San Francisco, CA | $250-999M | Census |
23 | ![]() | BI & Notebooks | San Francisco, CA | $250-999M | Hex |
24 | ![]() | Customer Data Analytics | San Francisco, CA | $250-999M | Hightouch |
25 | ![]() | Customer Data Analytics | Seattle, WA | $1B-$5B | Amperity |
26 | ![]() | Data Governance & Security | New York, NY | $1B-$5B | BigID |
27 | ![]() | Data Governance & Security | Fremont, CA | $250-999M | Privacera |
28 | ![]() | Data Governance & Security | Boston, MA | $250-999M | Immuta |
29 | ![]() | Data Observability | San Francisco, CA | $250-999M | Bigeye |
30 | ![]() | ELT & Orchestration | Greater Manchester, United Kingdom | $1B-$5B | Matillion |
31 | ![]() | Customer Data Analytics | San Francisco, CA | $1B-$5B | Heap |
32 | ![]() | AI/ML | San Francisco, CA | $250-999M | Tecton |
33 | ![]() | Query and Processing | Burlingame, CA | $250-999M | Imply |
34 | ![]() | BI & Notebooks | San Francisco, CA | $250-999M | Sisu Data |
35 | ![]() | ELT & Orchestration | San Francisco, CA | $100-$249M | Rudderstack |
36 | ![]() | Customer Data Analytics | New York, NY | $250-999M | ActionIQ |
37 | ![]() | Query and Processing | Portola Valley, CA | $1B-$5B | ClickHouse |
38 | ![]() | ELT & Orchestration | San Francisco, CA | $1B-$5B | Airbyte |
39 | ![]() | Query and Processing | San Mateo, CA | $250-999M | Rockset |
40 | ![]() | AI/ML | San Francisco, CA | $250-999M | Labelbox |
41 | ![]() | AI/ML | San Mateo, CA | $250-999M | Explorium |
42 | ![]() | AI/ML | San Francisco, CA | $100-$249M | Rasa |
43 | ![]() | ELT & Orchestration | Washington, DC | $250-999M | Prefect |
44 | ![]() | Query and Processing | New York, NY | $250-999M | Materialize |
45 | ![]() | AI/ML | New York, NY | $100-$249M | Coiled |
46 | ![]() | BI & Notebooks | San Mateo, CA | $100-$249M | Preset |
47 | ![]() | BI & Notebooks | San Francisco, CA | $100-$249M | Metabase |
48 | ![]() | AI/ML | San Francisco, CA | $100-$249M | Iterative.ai |
49 | ![]() | AI/ML | San Francisco, CA | $100-$249M | Robust Intelligence |
50 | ![]() | AI/ML | Mountain View, CA | $100-$249M | Fiddler |
Methodology
Data50 companies were founded after 2008, have raised new funding in the last two years, and their employee base is growing at at least 30% YoY. Their products are horizontal technologies serving data or data application teams across industries.
Rankings are based on a blend of most recent valuation, company size, employee growth over the last two years, years in operation, and current revenue scale. Employee data is based on publicly available data from LinkedIn. Funding data is based on publicly available data from Pitchbook and Crunchbase, and is accurate as of March 22, 2022.
Note that this list does not include transactional database companies such as CockroachDB, PlanetScale, and Yugabyte because usage of the data with those technologies is inherently transactional instead of analytical.
Looking under the covers, we’ve broken down the Data50 into seven subcategories.
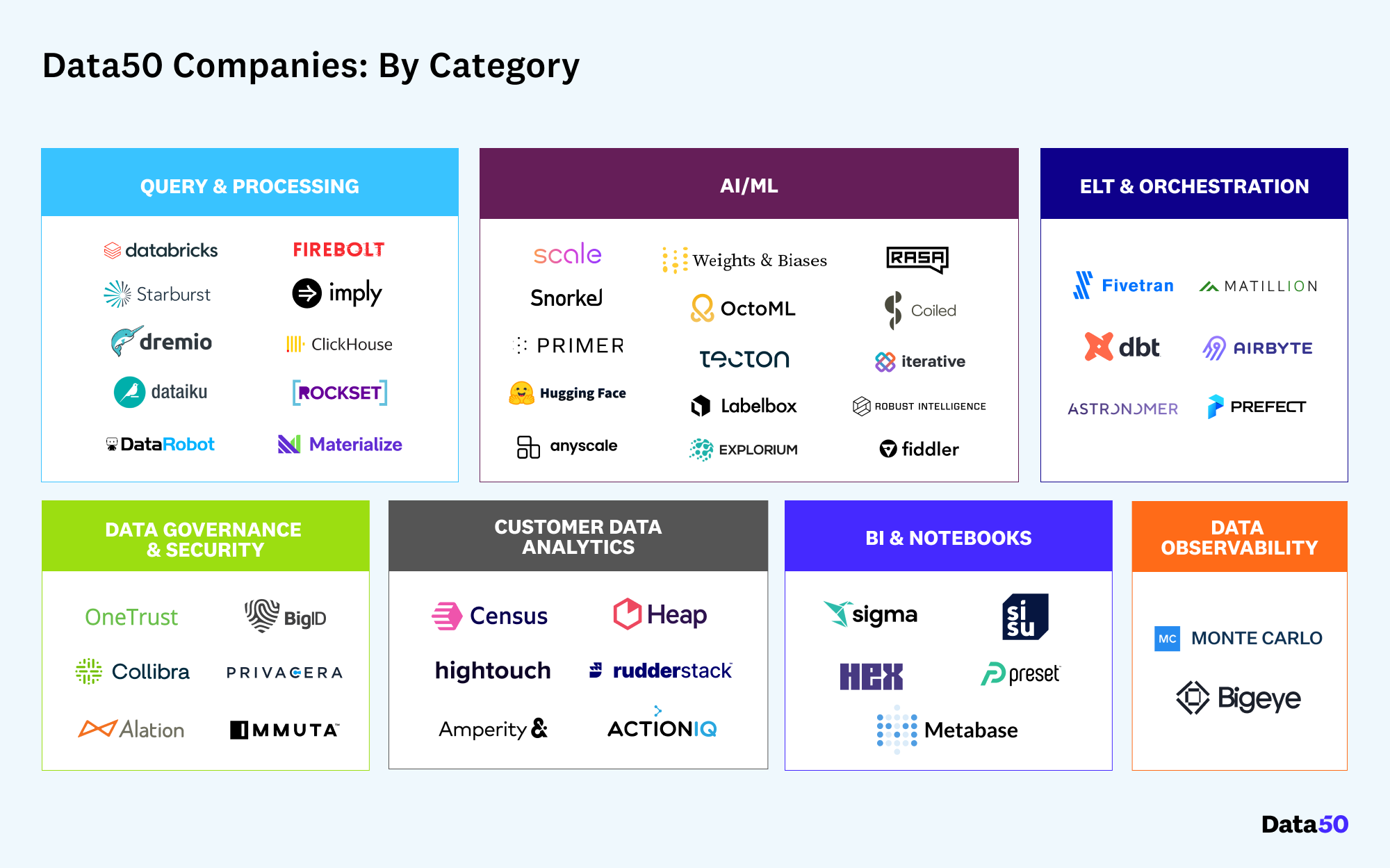
- Query and processing technology is the core engine to access, aggregate, and compute data. It involves two main classes: batch processing (e.g. Databricks and Starburst) and real-time processing (e.g. ClickHouse and Imply). The latter has been gaining more attention over the past few years, driven by increasing demand for real-time applications.
- AI/ML (artificial intelligence and machine learning) includes software that applies algorithmic modeling and machine learning for processing large scale data. This space is maturing and flourishing as evident from the sheer volume of companies that made the list. Some of the players are focused on a particular type of data (e.g. Rasa and Hugging Face for natural language), while others are focused on different areas, like the productization of AI (e.g. Scale, Tecton, and Weights and Biases) or acting as the “compute layer” for running AI workloads (e.g. Anyscale).
- ELT & orchestration enables the movement of data. It is the transportation layer that guarantees data arrives at its destination accurately and on time. This category evolved from the traditional ETL vendors that are built upon on-prem drag-and-drop interfaces. The new class of players, on the other hand, are mostly cloud-native (e.g. Fivetran and dbt), developer-friendly (e.g. Astronomer and Prefect), and handle more complex dependencies across different data environments.
- Data governance and security are becoming critical concerns as the data stack becomes increasingly complex and more stakeholders are involved. Governance tools are required — especially in highly regulated industries — to secure data and maintain compliance throughout the data lifecycle (e.g. OneTrust and Collibra). This category is relatively new and typically serves large enterprise companies that are under regulatory oversight.
- Customer data analytics has traditionally been owned by marketing teams. However, due to its increased importance, data teams are now more involved in integrating customer data with central data platforms. This category is focused on capturing customer data (e.g. Rudderstack and ActionIQ) or operationalizing that data to serve front-line business use cases (e.g. Census and Hightouch).
- BI & notebooks cover the consumption layer of data. Even though it is a well-established category, new players such as Preset or Metabase are taking an open source-first approach and appeal to technical data engineers, as well as business intelligence teams. The fast-changing nature of data needs also creates more demand for iterative and interactive notebooks (e.g. Hex) and automatic insight generation (e.g. Sisu).
- Data observability draws inspiration from best practices in the software engineering stack. As the data stack becomes increasingly interdependent on up and downstream tooling, and the accuracy of data has broader impact, observability emerged as the newest category to provide monitoring and diagnostic capability across the data flow.
Even though the main market tailwind driving adoption is the increasing volume and usage of data, the underlying drivers differ for each category. For example, the advances happening in the querying and processing space are mainly driven by the separation of compute and storage, movement to the cloud, and cheaper computing power. Meanwhile, the adoption of operational tooling in data governance and data observability is largely driven by the growing operational use cases and complexity of data workflows.
Query and processing companies have raised the lion’s share of capital
The query and processing category only accounts for one-fifth of the companies in Data50, but the amount of capital — almost 50% of all funding — invested in this category is staggering. Even though this data is influenced by Databricks’s recent $1.6B funding round, the category would still account for 37% of all funding — more than twice that of the next category — without it.
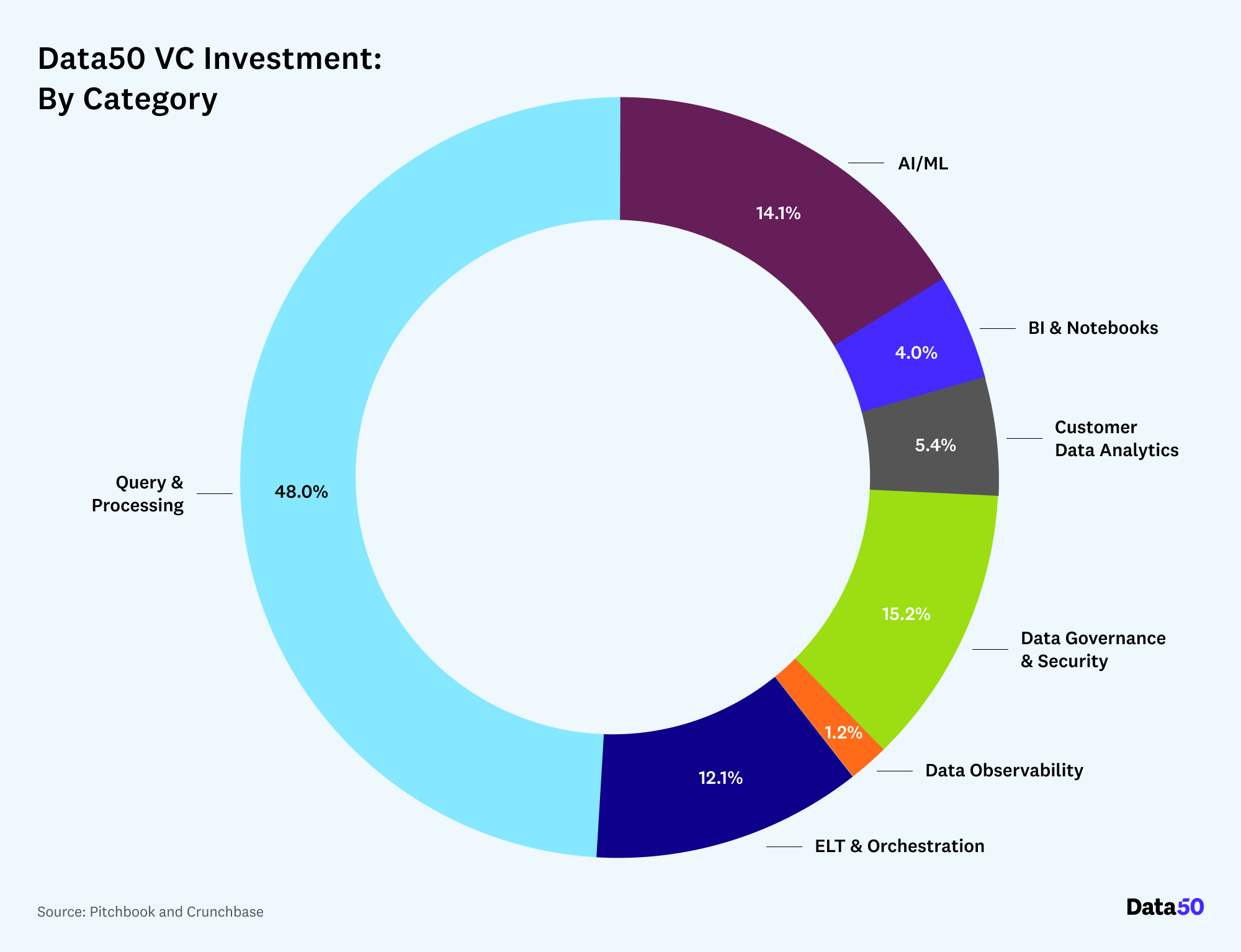
When looking at the categories by company count, the distribution is more balanced. AI/ML is the biggest category by the number of companies, largely because the space is still evolving and requires a new separate set of tools to train, measure, and productionize models. (For more on how this space is evolving, read Emerging Architectures for Modern Data Infrastructure.)
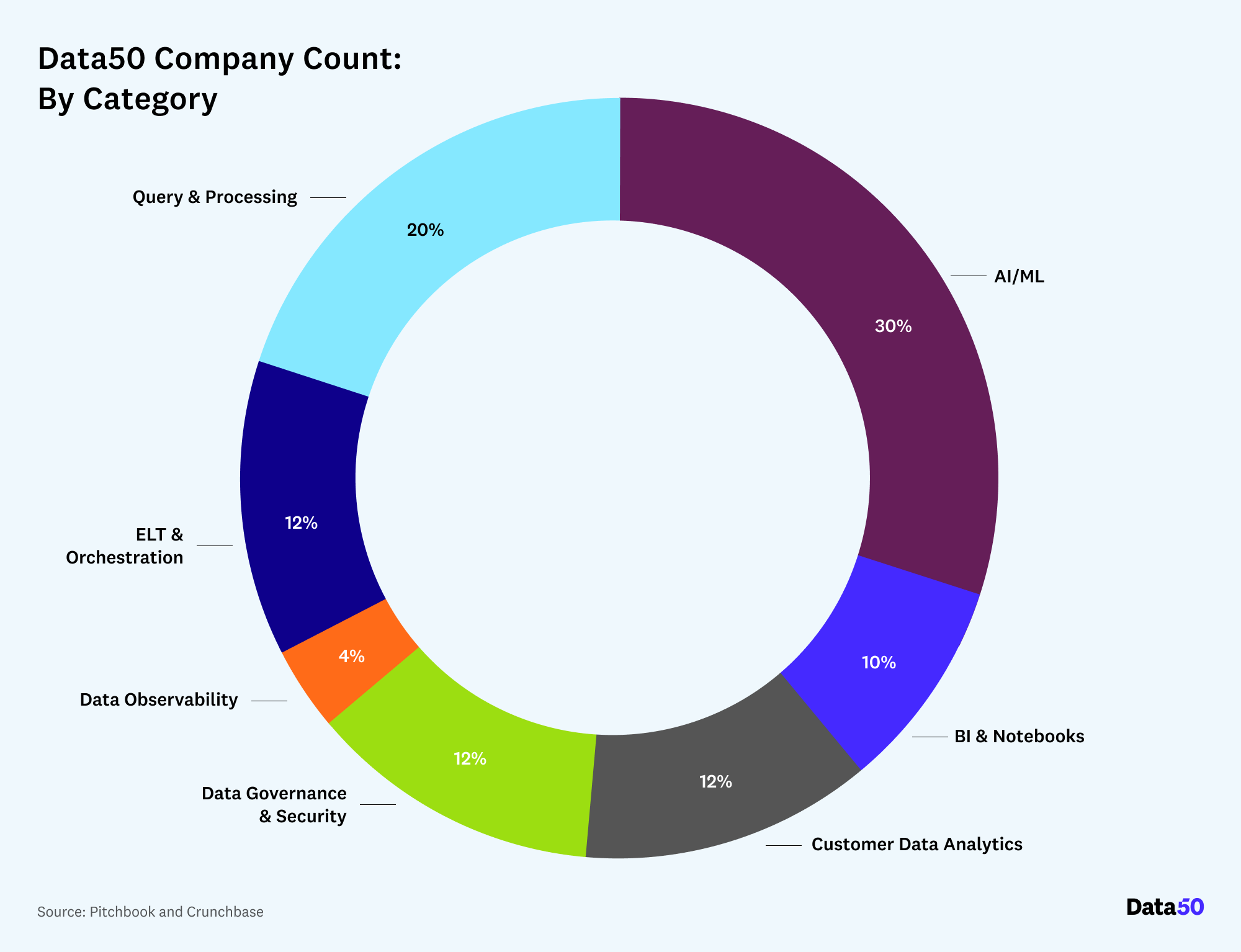
The Data50 is clustered in the Bay Area
Of the 50 companies, 47 (94%) are based in the United States and three are international. The majority of the companies, 33, are based in the San Francisco Bay Area, while nine are along the I-95 corridor in Washington, D.C., Philadelphia, New York, and Boston. Two are based in Seattle, one is based in Cincinnati, and one is based in Atlanta.
Such distribution is heavily impacted by where the large-scale data ecosystem resides historically (Oracle and Teradata were both founded in the Bay Area, for example). However, we’re seeing more data companies popping up across the globe (e.g. Firebolt and Matillion) as data engineering talent and demand for data tooling reach nearly every continent.
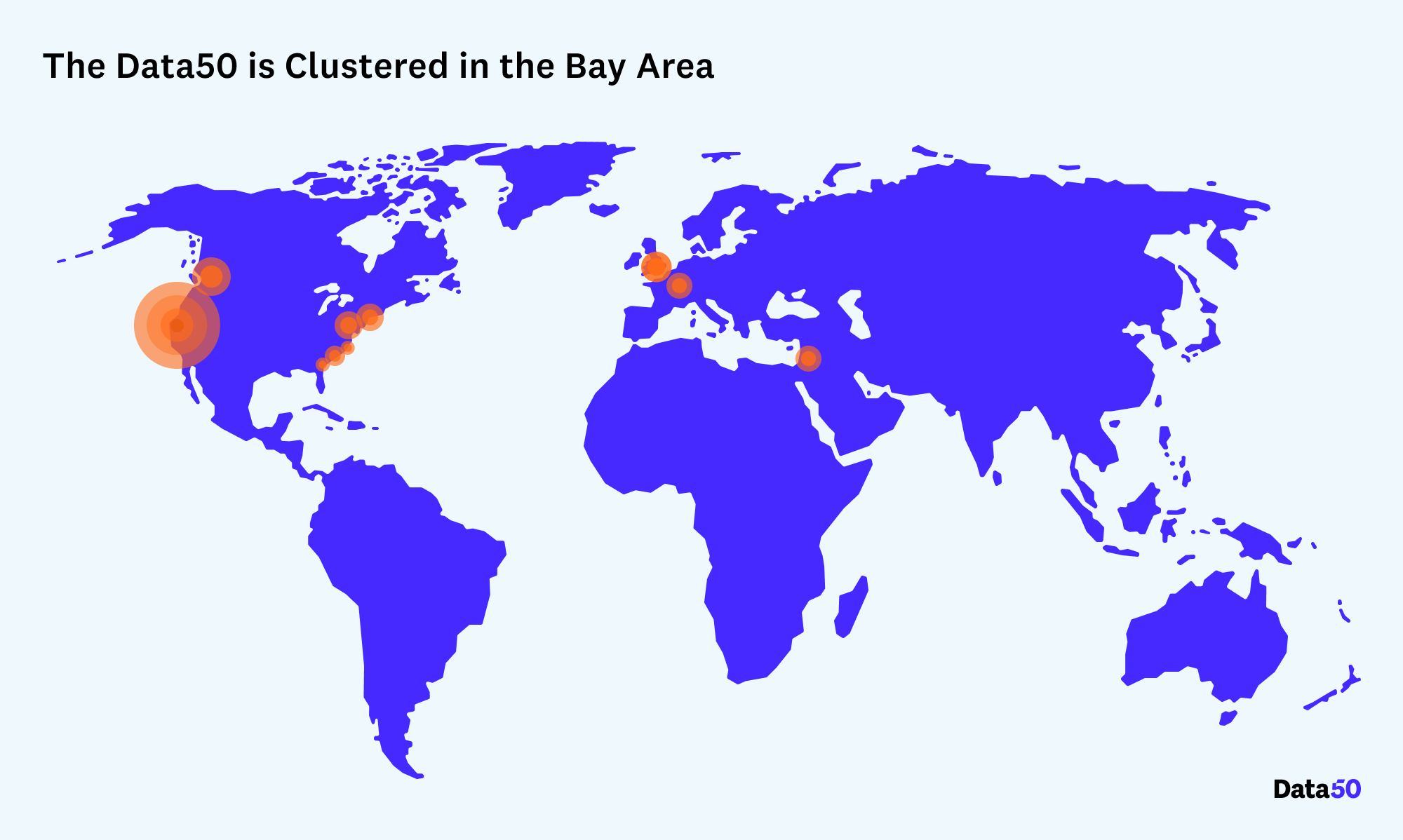
AI/ML category drove spike of new data companies in 2019
The majority of the Data50 companies were founded after 2014, with a peak around 2019, driven by the explosion of AI/ML tooling. In fact, many more data companies were founded after 2019, but because we’re focused on companies that have reached a certain scale, most newer companies don’t appear on this list yet.
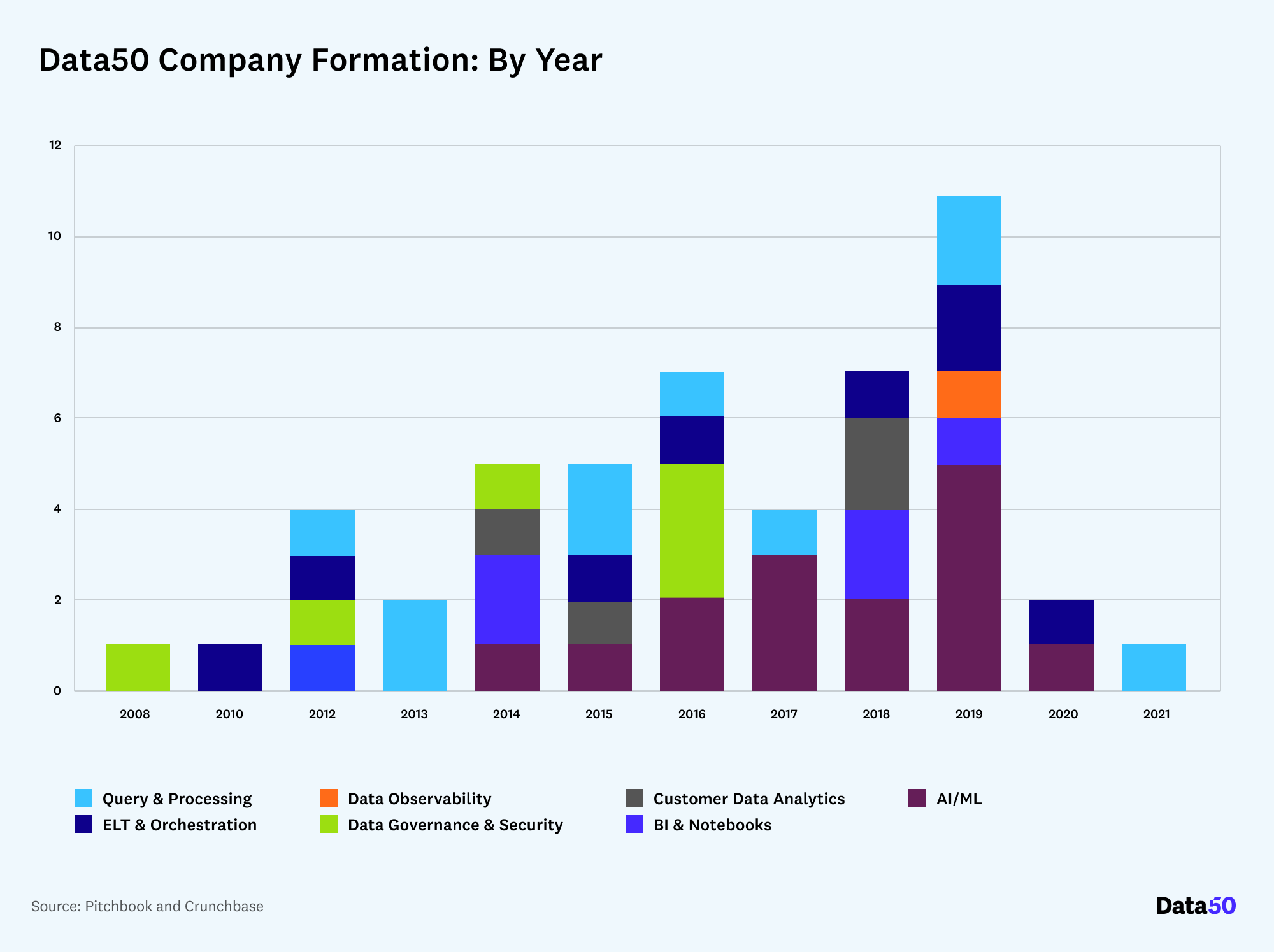
Investment dollars are growing in every category
Looking at per category investment, the most notable trend is that AI/ML companies are picking up more investor interest than ever, mostly concentrated in the early stage. The same holds true for ELT and orchestration – largely driven by mega rounds from Fivetran and dbt. Query and processing companies continue to attract big dollars, although the companies tend to be in the later stage.
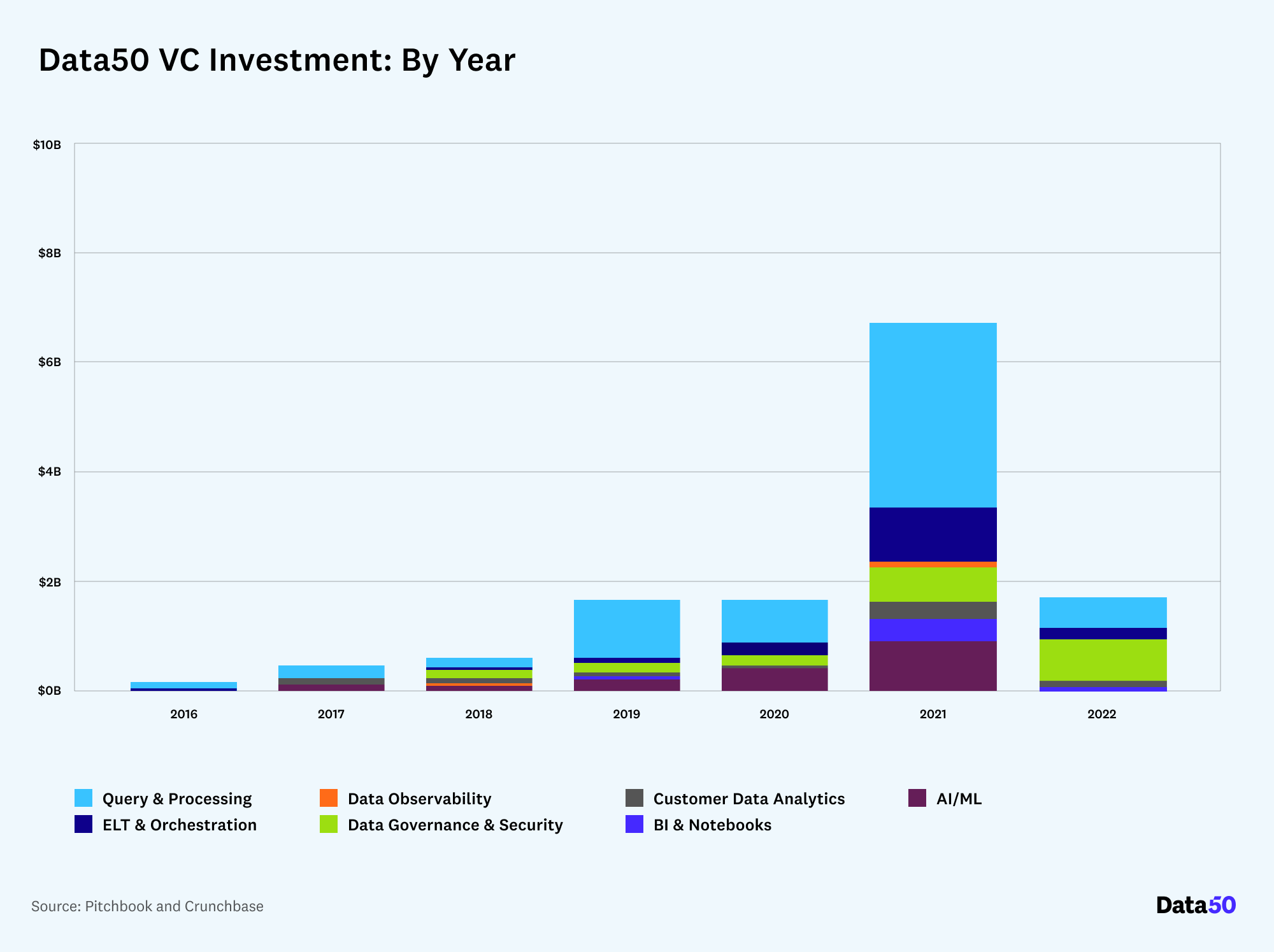
We firmly believe the next 10 years will be the decade of data, encompassing infrastructure, applications, and everything in between. As a result, we’ll continue to see record-breaking growth, funding, and market capitalization, which we will track annually in this list. Congratulations to all the companies in the first Data50 class!
Views expressed in “posts” (including articles, podcasts, videos, and social media) are those of the individuals quoted therein and are not necessarily the views of AH Capital Management, L.L.C. (“a16z”) or its respective affiliates. Certain information contained in here has been obtained from third-party sources, including from portfolio companies of funds managed by a16z. While taken from sources believed to be reliable, a16z has not independently verified such information and makes no representations about the enduring accuracy of the information or its appropriateness for a given situation.
This content is provided for informational purposes only, and should not be relied upon as legal, business, investment, or tax advice. You should consult your own advisers as to those matters. References to any securities or digital assets are for illustrative purposes only, and do not constitute an investment recommendation or offer to provide investment advisory services. Furthermore, this content is not directed at nor intended for use by any investors or prospective investors, and may not under any circumstances be relied upon when making a decision to invest in any fund managed by a16z. (An offering to invest in an a16z fund will be made only by the private placement memorandum, subscription agreement, and other relevant documentation of any such fund and should be read in their entirety.) Any investments or portfolio companies mentioned, referred to, or described are not representative of all investments in vehicles managed by a16z, and there can be no assurance that the investments will be profitable or that other investments made in the future will have similar characteristics or results. A list of investments made by funds managed by Andreessen Horowitz (excluding investments for which the issuer has not provided permission for a16z to disclose publicly as well as unannounced investments in publicly traded digital assets) is available at https://a16z.com/investments/.
Charts and graphs provided within are for informational purposes solely and should not be relied upon when making any investment decision. Past performance is not indicative of future results. The content speaks only as of the date indicated. Any projections, estimates, forecasts, targets, prospects, and/or opinions expressed in these materials are subject to change without notice and may differ or be contrary to opinions expressed by others. Please see https://a16z.com/disclosures for additional important information.